To Do
✅ python-pcl 설치
✅ 라이브러리 활용해보기
python-pcl 설치
하 여러번 시도 끝에 드디어 이 라이브러리를 쓸 수 있는 환경을 만들었다.
아마 이 포스트를 보는 사람들 중에 개고생한 사람들 여럿 있지 않을까 싶다.
docker로 빌드는 됐었지만 익숙치 않다보니 다시 설치를 시도해봤다.
설치 성공 경험을 다음과 같이 공유한다.
아래 중국 친구가 csdn에서 설치 경험을 공유해줬는데 나도 성공했다.
설치 세팅이 완료되면, 시각화에 조금 용이한 open3d 라이브러리를 설치해줘야하는데
pip install, conda install 둘 다 안돼서, 다음의 명령어로 설치했다.
conda install -c open3d-admin -c conda-forge open3d
관련해서 유용했던 링크들도 아래에 남겨두겠다.
[ Package Github 링크 및 참고 유용 사이트 ]
https://github.com/strawlab/python-pcl
GitHub - strawlab/python-pcl: Python bindings to the pointcloud library (pcl)
Python bindings to the pointcloud library (pcl). Contribute to strawlab/python-pcl development by creating an account on GitHub.
github.com
https://github.com/adioshun/gitBook_Tutorial_PCL
GitHub - adioshun/gitBook_Tutorial_PCL: PCL Library Tutorial 작성 중
PCL Library Tutorial 작성 중 . Contribute to adioshun/gitBook_Tutorial_PCL development by creating an account on GitHub.
github.com
https://dreamfuture.tistory.com/51
python PCL 사용하기( Windows 10 with PIP)
1. 개요 Point Cloud Library(PCL)는 point cloud 데이터를 처리하는 open-source library이다. 윈도우즈 환경에서 파이썬을 이용하여 PCL 라이브러리를 pip로 설치하고 사용한다. https://pointclouds.org/ 2. OpenNI 설치 Po
dreamfuture.tistory.com
라이브러리 활용해보기
.env에 경로 관리를 하는 경우가 많아서 dotenv도 import해뒀다.
여기 예제에서는 이용하지는 않았다.
[ Pointcloud normal compute ]
import pcl
import numpy as np
import open3d as o3d
from dotenv import load_dotenv
import matplotlib.pyplot as plt
# Pointcloud normals compute
def get_normals(cloud, Radius=True):
"""
Parameters
----------
cloud : pcl.PointCloud
Returns normals
-------
"""
feature = cloud.make_NormalEstimation()
if Radius:
feature.set_RadiusSearch(0.03) #Use all neighbors in a radius of input
normals = feature.compute()
else: # KSearch
tree = cloud.make_kdtree()
feature.set_SearchMethod(tree)
feature.set_KSearch(10)
normals = feature.compute()
return normals
# Pointcloud 불러오기
p = pcl.load("./samples/table_scene_lms400.pcd")
# Array로 다루고자 하면 변환
p_array = p.to_array()
# get_normals fn 사용 (default: RadiusSearch)
normals = get_normals(p)
[ Visualization with Open3d ] - version 0.3.0
open3d 0.3.0 버전에서는 class 구조가 다르게 작성되어 있는 것 같은데,
다음의 코드로 시각화 할 수 있다.
pcd = o3d.PointCloud()
pcd.points = o3d.Vector3dVector(p_array)
o3d.draw_geometris([pcd])
실제로 compute된 normals가 어떤지 보기 위해서 open3d를 이용해보려고 했으나,
설치한 가상환경에서는 compatibility가 open3d 0.3.0 버전밖에 안맞는지 설치가 상위 버전이 안된다.
더 상위 버전을 사용하고자 함은, draw_geometries 메소드 내 point_show_normal 이 option에 없었기 때문이다.
가상환경 깨질까봐 더 못만지겠고 예전에 깔아줬던 가상환경 안에서 open3d 0.13.0로 시도했다.
즉, 다음의 상황:
일단, compute 된 normals를 포함하여 x, y, z, nx, ny, nz를 .pcd 형식의 파일로 write해주고(pcl 환경)
다시 읽어들였다(open3d 환경).
[ Write files with Open3d ] - version 0.3.0
pcd.points = o3d.Vector3dVector(p.to_array())
pcd.normals = o3d.Vector3dVector(get_normals(p).to_array()[:,:3])
o3d.write_point_cloud("table_scene_lms400_normals.pcd", pcd)
위와 같이 normal을 포함하여 .pcd 형식의 파일로 export를 해준다.
[ Read files and visualize with Open3d ] - version 0.13.0
pcd = o3d.io.read_point_cloud(r"파일경로/table_scene_lms400_normals.pcd")
o3d.visualization.draw_geometries([pcd], point_show_normal=True)
open3d 0.13.0 에서는 위에 0.3.0 문법이랑 사알짝 다르다.
결과는 다음과 같다:
위에랑 같은 view로 맞춰줄수도 있었지만 귀찮다. 몇줄 적는것도 귀찮아서야 원...
필요하면 open3d docs를 살펴보면 될 것 같다.
http://www.open3d.org/docs/0.13.0/
Open3D: A Modern Library for 3D Data Processing — Open3D 0.13.0 documentation
© Copyright 2018 - 2020, www.open3d.org
www.open3d.org
http://pointclouds.org/documentation/tutorials/
Introduction — Point Cloud Library 1.13.0-dev documentation
Title: The PCL Registration API Author: Dirk Holz, Radu B. Rusu, Jochen Sprickerhof Compatibility: > PCL 1.5 In this document, we describe the point cloud registration API and its modules: the estimation and rejection of point correspondences, and the esti
pointclouds.org
다음 post에서는 좀 더 재밌는 segmentation에 관련해서 작성해보겠다.
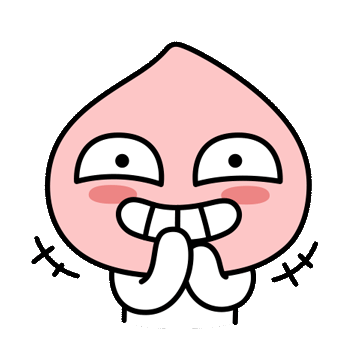
'Researches > Pointclouds' 카테고리의 다른 글
[Python] 3차원 영역확장 알고리즘 (3D region growing algorithm) (0) | 2023.02.14 |
---|---|
pointcloud 머신러닝/AI 트레이닝 데이터셋 전처리(Pre-processing) (0) | 2022.06.23 |